What are the manufacturing challenges faced by 2.5D IC packaging?
The packaging of artificial intelligence chips is like a puzzle composed of individual blocks of varying sizes and shapes, each of which is crucial to the final product. These devices are typically integrated into 2.5D IC packaging, aimed at reducing the space occupied and maximizing bandwidth.
Graphics Processing Units (GPUs) and multiple 3D High Bandwidth Memory (HBM) stacks form the main part of the AI puzzle. Advanced IC substrates (AICS) lay the foundation for building 2.5D packaging.
In this article, we will focus on the advanced packaging aspects of the process, as well as the manufacturing challenges faced in 2.5D IC packaging.
But before discussing this issue, let's talk about what artificial intelligence is.
About Artificial Intelligence
As is well known, artificial intelligence is just a new type of technological tool. Its function is the same as that of other tools: to enable users to complete tasks more efficiently and easily. Below is a list of different types of artificial intelligence, which happens to be created using generative artificial intelligence.Artificial Intelligence (AI): This is a broad term for technology that simulates human intelligence, enabling machines to learn, think, and make decisions like humans, thereby being able to autonomously perform various tasks.
Advertisement
Generative Artificial Intelligence (GAI): Generative AI is a type of artificial intelligence that can be used to create new content and ideas, including conversations, stories, images, videos, and music. AI technology attempts to mimic human intelligence in non-traditional computing tasks such as image recognition, natural language processing (NLP), and translation.
Machine Learning (ML): This is a branch of artificial intelligence that enables computers to "self-learn" from training data and improve over time without explicit programming. Machine learning algorithms can detect patterns in data and learn from them in order to make their own predictions. In short, machine learning algorithms and models learn from experience.
Deep Learning: This is a subfield of machine learning that uses artificial neural networks to mimic the learning process of the human brain. It focuses on neural networks to solve complex problems.
Each of the above applications benefits from or requires high-performance computing capabilities.
Now that we have discussed artificial intelligence, let's further explore the packaging challenges of 2.5D AI chips. This article will focus on challenges related to Through-Silicon Vias (TSVs), microbumps, and AICS.
TSV Challenges
TSVs are a key part of the manufacturing and performance challenges of 2.5D and 3D packaging. TSVs have extremely small critical dimensions, high aspect ratios (HAR), and fine pitches, enabling a large number of input/output connections and providing vertical electrical pathways for HBM and silicon interposers.
The TSV process is dense and requires several key process steps, including etching, deposition, filling, and chemical-mechanical planarization (CMP). As the demand for thinner silicon chips increases, reducing TSV sizes, and in some cases, higher aspect ratios, controlling precise dimensions and depths, and uncovering an increasing number of hidden defects, are key to maintaining high yields.The critical dimensions at the top and bottom (CD), side wall profiles, and depths are all important process control parameters in TSV manufacturing, as they affect the electrical performance between stacked chips. If the TSV etching is not deep enough, even if two dies are placed on top of each other, they will not be connected. Next, a barrier layer/liner material with good uniformity and thickness control is deposited. Copper electroplating fills the TSV, where measuring the coverage layer thickness and inspecting the growth defects and voids of copper filling is crucial.
As for the backside of the wafer, the front side of the wafer will be temporarily bonded to a carrier, so the backside can be thinned to expose the TSV. The thinning process is important. It is necessary to measure and monitor the remaining silicon of the etched TSV for grinding and blanket etching to ensure that the TSV interconnections are uniformly exposed for stacking chips or the entire wafer. Failure to accurately measure and inspect the backside may lead to defects, deformation, resistance, and device failures, ultimately resulting in increased scrap and reduced yield.
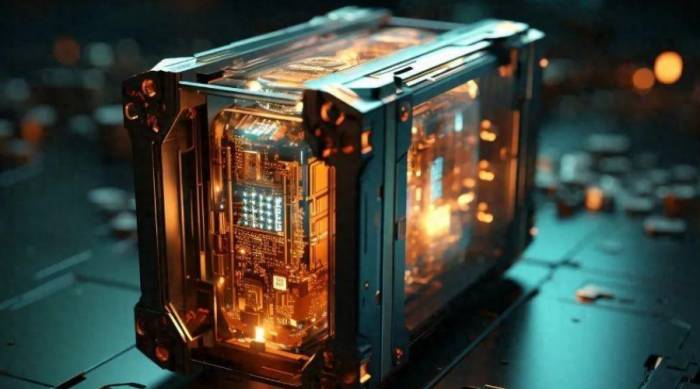
Tools that help address the above challenges include metrology for advanced OCD and HAR structures, as well as automated high-speed sub-micron defect detection and 2D/3D metrology systems.
**Microbump Challenges**
In addition to TSVs, microbumps are also key elements that provide interconnections between different components within an AI package. In addition to connecting the various DRAM layers and logic buffer chips within an HBM stack, microbumps also connect the 3D memory stack and GPU to the interposer layer. Larger solder bumps also connect the interposer layer to the advanced IC substrate (AICS).
Very similar to TSVs, microbump technology continues to scale down, reducing height, diameter, and pitch. Further scaling down is expected, and eventually, direct copper-copper hybrid bonding will be required. The main disadvantage of this shrinkage is maintaining the uniformity of bump plating — both within the chip and across the entire wafer. This becomes more challenging. To properly connect the chip to the next component (whether it be DRAM, logic buffer chips, interposer layer, or IC substrate), these bumps need to have the same height to ensure proper connection.
Measuring the individual thickness of each metal film used to construct the bumps is also important. The choice of metal and their respective thicknesses is crucial for controlling the performance and reliability of the device.
Another potential obstacle with microbumps is related to defects: the presence of residues, cracks, voids, or, to a greater extent, microbumps being damaged or displaced. In extreme cases, these defects can lead to immediate electrical short circuits or connection failures. However, the impact of some of these defects may not be apparent at first but can slowly develop and affect the reliability of the device.
If not properly addressed, each of these challenges will affect device performance. Photoacoustic metrology tools using picosecond ultrasound technology can measure the thickness of individual metal films and the final total bump height. A combination of 2D/3D metrology and inspection tools can measure bump diameter and bump height, as well as detect defects, providing in-line process control.AICS Challenge
As the density of input/output (I/O) increases, the ability of individual components to mate directly with the printed circuit board becomes an issue. AICS acts as a bridge between various components of the software package. To connect the intermediary layer above and the chips connected to it, a significant amount of redistribution layers (RDLs) is required. With the increase in the number of RDL layers, the possibility of overlay errors also increases.
Speaking of RDLs, the large solder pads at the end of each interconnect line/space (L/S) are connected to vias. The landing pads are significantly larger than the critical dimension of the RDL. This helps to improve the overlay tolerance. However, these large landing platforms limit the design space. As interconnect technology demands finer L/S, this problem will only become more severe. This leads to the need to increase the number of RDL layers, thereby increasing costs and potential yield loss.
To alleviate this design dilemma, smaller RDL landing pads are needed. This can be achieved if the process coverage is improved. To achieve this goal, the lithography system must analyze and compensate for the deformation errors caused by the repeated thermal cycling of the copper clad laminate (CCL) panel and dielectric during the entire build process. Accurate metrology data is needed to generate the best alignment solution. However, this data is typically available after the lithography process is completed and the coverage of the via to the RDL joint solder pad is measured. It is crucial to analyze this overlay data and feedback the corrections back to the stepper to compensate for panel distortion in future panels.
Another area of concern involves the uniqueness of the AICS process. For wafer-based devices, the active circuit structures occur only on one side of the wafer. But for AICS, both the front and back sides of the panel are processed. This significantly increases the risk of yield loss due to defects caused by surface contamination. Moreover, the number of packages per AICS panel is relatively small. For example, a 510mm x 515mm AICS panel can only accommodate 16 packages (120mm x 120mm), while a fan-out panel-level packaging (FOPLP) can accommodate over 2,300 packages. In other words, a defective package on AICS could lead to a 6.25% yield loss, while for FOPLP, a defective package could lead to a 0.04% yield loss. As the AICS package size increases to 150mm x 150mm, the yield challenge becomes even more severe: a single defective package failure could lead to an 11% yield loss.
Plating, dry film resist and build-up film non-uniformity, RDL line defects, and more subtle buried defects (such as bubbles and particles under the lamination) all lead to yield loss. More stringent process control through metrology measurements and inspections after each critical step alerts manufacturers to potential process deviations so that corrective actions can be taken immediately. AICS manufacturing is a lengthy process, taking several weeks to process both sides of the panel. Therefore, real-time tracking of the yield of each layer can help reduce the time spent on processing defective substrates.
Conclusion
Advanced packaging is just a small part of the artificial intelligence puzzle, but in this post-Moore era, the backend of the process is more important than ever. In this article, we outlined several key challenges faced by advanced packaging for artificial intelligence devices, from measuring CD and identifying defects related to TSVs and microbumps, to real-time tracking of the detection packages in the AICS production process. As the artificial intelligence market drives the growth of the current semiconductor industry, the solutions described here will become a key part of solving the puzzle of how to meet the rapidly growing demand for artificial intelligence packaging.Please provide the text you would like translated into English.
Comment